
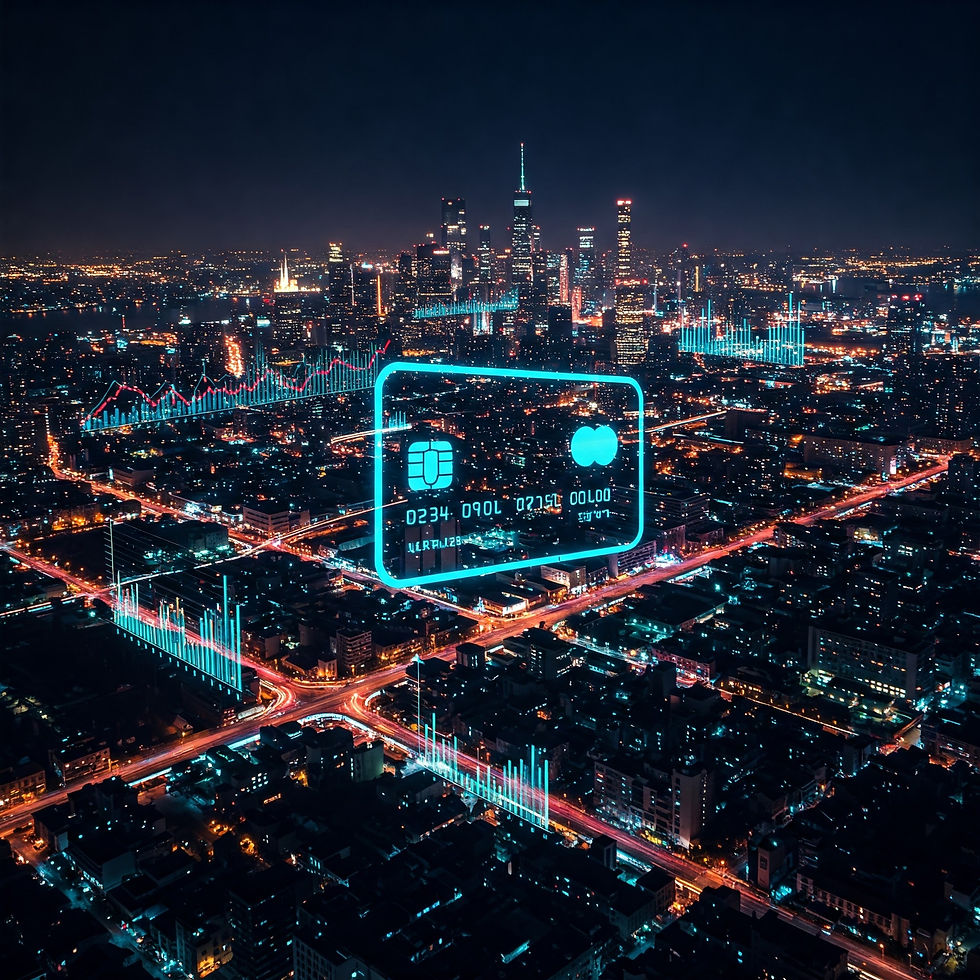
[ML] Credit Card Default Prediction
Develop a predictive model using customer demographic and transaction data to identify potential credit card defaulters, improving financial liquidity and risk management.
Objective
Predict potential credit card defaulters using customer demographic and transaction data.
Develop a model to proactively manage high-risk customers and improve financial liquidity.
Dataset Overview
Source: [UCI Machine Learning Repository]
Description: Binary classification of default occurrences (1: default, 0: non-default).
Total Records: 26,457
Number of Features: 20
Problem Definition
Challenge: Rising defaults due to aggressive marketing increasing new card issuances.
Risk: Delayed recovery of credit card dues leading to liquidity issues.
Expected Impact
Increase in financial liquidity through efficient recovery from defaulters.
Leverage the default prediction model for customer management programs and marketing campaigns.
Approach
Data Collection and Preparation
Collect and preprocess customer data, including credit card usage history and demographic information.
Model Development
Utilize recent transaction history and customer features to train the prediction model.
Focus on binary classification to identify high-risk customers.
Model Deployment and Operations
Implement the model for monthly extraction of high-risk customers.
Use predictions to manage defaulters and run targeted marketing campaigns.
Key Code Highlights
Handle missing values (df['occyp_type'].fillna('etc')).
Process credit labels and transform age bins (optb.transform(df['DAYS_BIRTH'], metric="bins")).
Practical Application
Use model predictions to enhance liquidity by managing defaulters effectively.
Increase revenue through targeted marketing for high-risk customers (e.g., additional products, revolving credit).